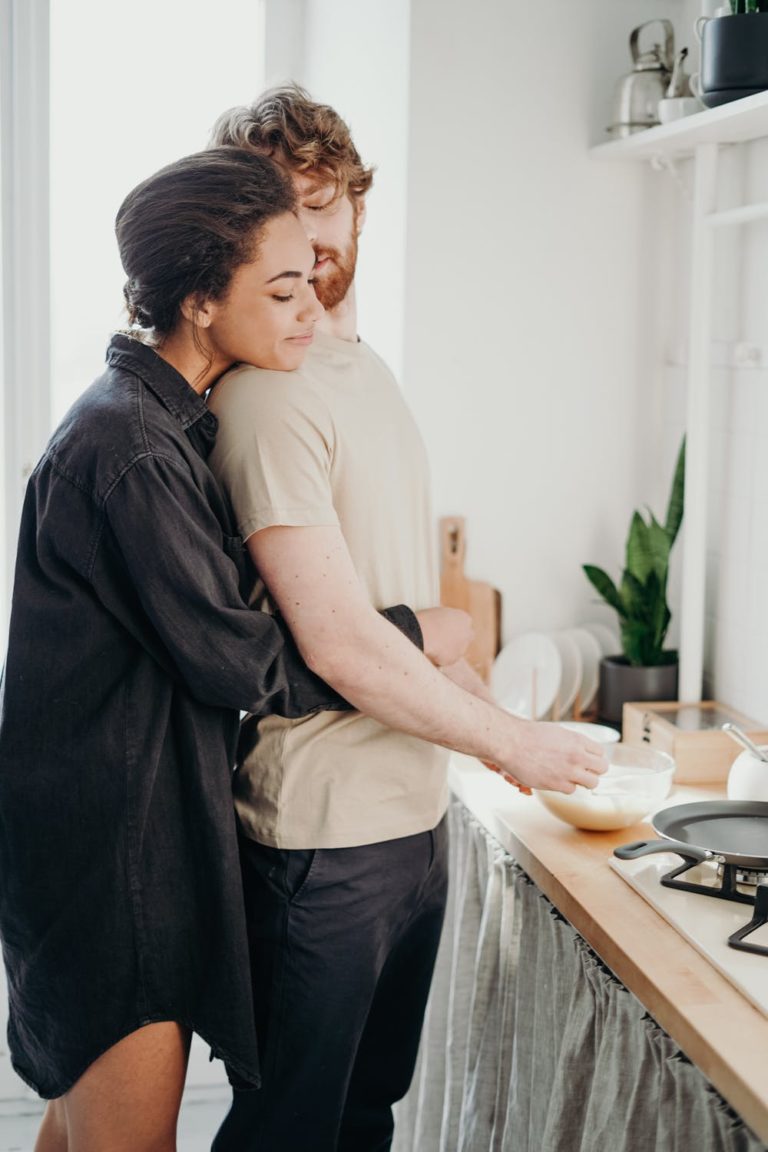
Restaurants are a great business to run if you are an enterprising business person. On one hand, people don’t show any signs of not eating anytime soon. They will always want to head out to a restaurant for a variety of reasons. Sometimes, people just don’t feel like cooking. Restaurants can also become meeting places
With the prevalence of Oprah’s book club, it’s no surprise that many readers turn to Oprah Winfrey to find additions
After two years of living through a public health emergency, it’s no surprise that many people are thinking more about
There has been an increase in interest connected to careers in health care lately, and for good reason. There are
Gemstones in every color of the rainbow are loved by people all over the world. Whether for their meanings or